Tech News
Gemini’s data-analyzing abilities aren’t as good as Google claims
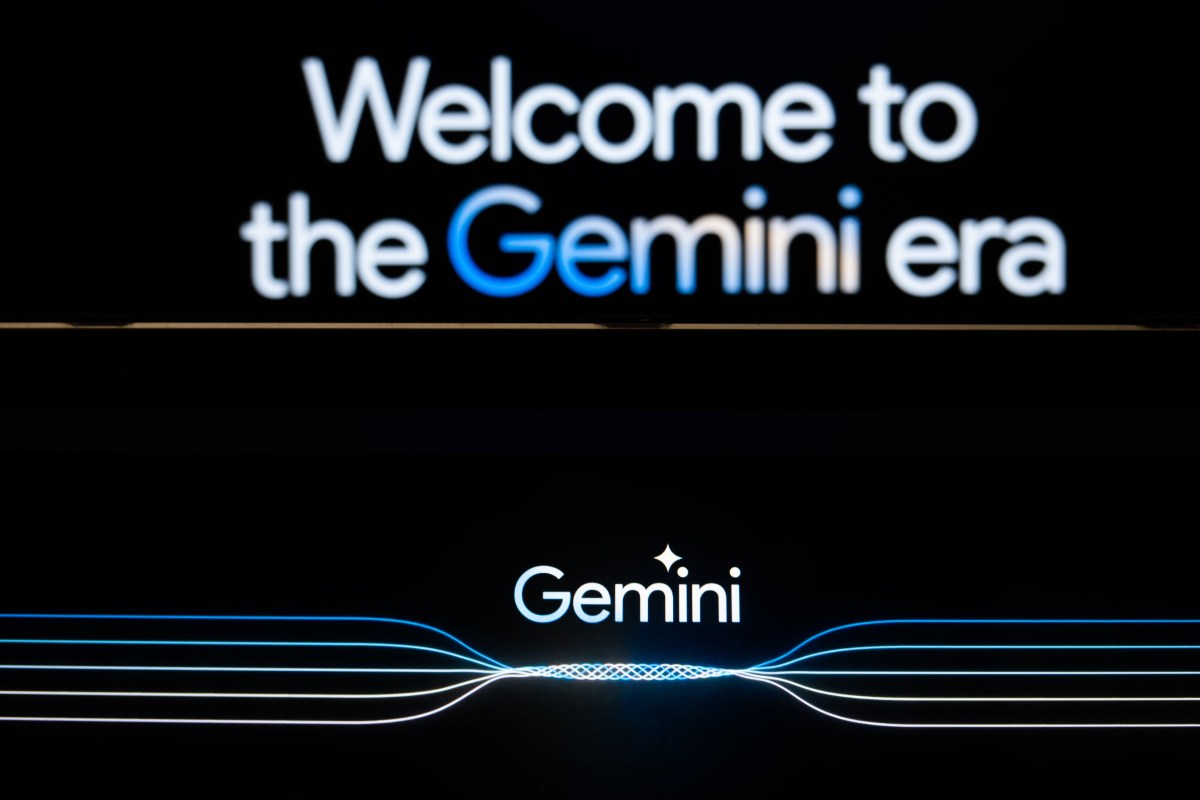
Google’s flagship generative AI models, Gemini 1.5 Pro and 1.5 Flash, are touted for their ability to process large amounts of data. However, recent research suggests that these models may not be as effective as claimed.
Two separate studies found that Gemini 1.5 Pro and 1.5 Flash struggle to accurately answer questions about large datasets, with correct answers given only 40-50% of the time in some tests.
Researchers have observed that while these models can technically process long contexts, they may not truly understand the content they are analyzing.
Gemini’s context window is lacking
Gemini’s context window refers to the amount of input data it considers before generating output. The latest versions of Gemini can handle up to 2 million tokens as context, the largest of any commercially available model.
Despite demonstrations highlighting Gemini’s long-context capabilities, research has shown that the models struggle to accurately evaluate true/false statements about complex works of fiction.
In another study, Gemini 1.5 Flash performed poorly in reasoning tasks involving video content, suggesting limitations in its ability to understand and analyze visual data.
Google is overpromising with Gemini
While the studies have not been peer-reviewed and tested older versions of Gemini models, they raise concerns about Google’s claims regarding the capabilities of these AI models. Other models tested in the studies also showed poor performance in similar tasks.
Google is the only model provider that prioritizes context window in its advertisements, according to Saxon. While the technical details of models are important, the real question is how useful they are in practical applications.
Generative AI is facing increased scrutiny as businesses and investors question its limitations. Recent surveys show that many C-suite executives doubt generative AI’s ability to boost productivity and are concerned about potential errors and data breaches. Deal-making in generative AI has also declined in recent quarters.
Customers are seeking innovative solutions amidst chatbots that provide inaccurate information and AI search platforms that generate plagiarized content. Google tried to differentiate itself with Gemini’s context feature, but it may have been too early.
There is a lack of transparency in how models handle long context processing, making it difficult to verify claims of reasoning and understanding. Without standardized benchmarks and third-party evaluation, it is challenging to assess the true capabilities of generative AI models.
Google did not provide a comment on these issues. Saxon and Karpinska suggest that better benchmarks and more independent scrutiny are needed to address exaggerated claims about generative AI. Current benchmarking methods may not accurately measure a model’s ability to answer complex questions, leading to potential misconceptions about their capabilities.
-
Destination7 months ago
Singapore Airlines CEO set to join board of Air India, BA News, BA
-
Breaking News8 months ago
Croatia to reintroduce compulsory military draft as regional tensions soar
-
Tech News11 months ago
Bangladeshi police agents accused of selling citizens’ personal information on Telegram
-
Breaking News9 months ago
Bangladesh crisis: Refaat Ahmed sworn in as Bangladesh’s new chief justice
-
Guides & Tips9 months ago
Have Unlimited Korean Food at MANY Unlimited Topokki!
-
Gaming8 months ago
The Criterion Collection announces November 2024 releases, Seven Samurai 4K and more
-
Toys10 months ago
15 of the Best Trike & Tricycles Mums Recommend
-
Productivity10 months ago
How Your Contact Center Can Become A Customer Engagement Center