Gadgets
AI trained on AI churns out gibberish garbage
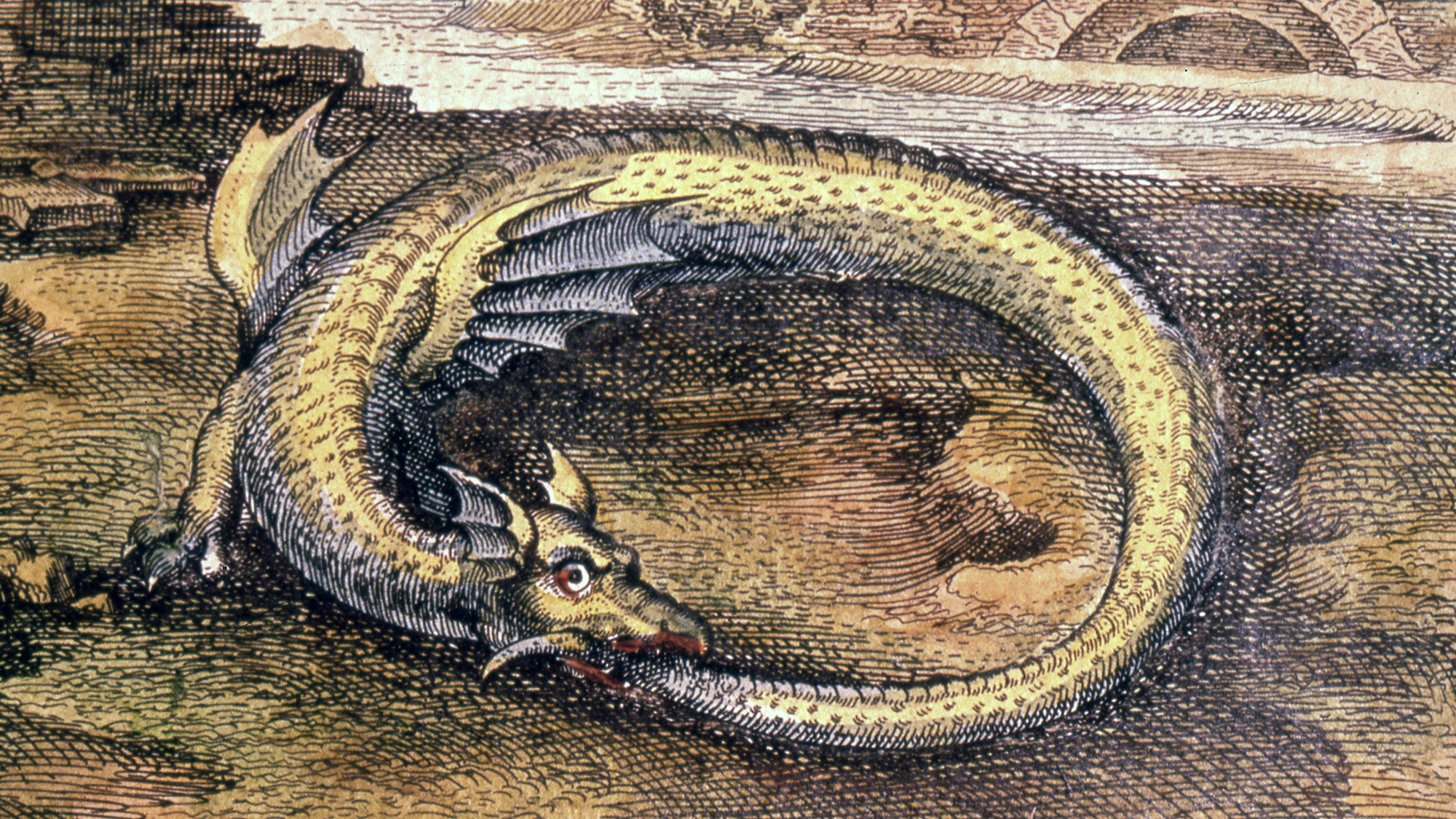
Large language models such as those developed by OpenAI and Google are known to require extensive training data to function effectively. With the latest iterations having already combed through a significant portion of the existing internet, concerns have arisen regarding the availability of new data to train future models. Meta CEO Mark Zuckerberg and others in the industry have suggested a potential solution to this data scarcity issue: training new AI systems on outputs generated by older AI models.
However, recent research indicates that relying on past model outputs for training could lead to a phenomenon known as “model collapse,” resulting in incoherent AI-generated content. In a study, researchers observed a degradation in the quality of AI-generated text over several generations, culminating in nonsensical outputs. This process, described as “becoming poisoned with its own projection of reality,” highlights the potential pitfalls of training AI models on their own outputs.
AI models forget meaning the more they trains on themselves
The concept of model collapse involves a progressive deterioration of AI models as they are trained on successive generations of their own outputs. Early signs of collapse manifest as the models begin to overlook outliers and unique elements in the original training data, leading to a homogenization of outputs. This lack of diversity could result in a skewed representation of reality, with minority perspectives being marginalized.
In later stages of collapse, the models lose touch with the original training data, generating incomprehensible gibberish. This indiscriminate self-cannibalization of previous outputs creates irreversible defects in the final model, signaling a breakdown in the learning process.
The researchers emphasize that this cascading effect and eventual collapse are inevitable for large models trained on their own data, particularly in the context of language models. While the study focuses on language models, the implications for multimodal models like image and video generators remain unclear.
Preserving original human text could stave off collapse
To mitigate the risk of model collapse and the proliferation of AI-generated content in training sets, researchers propose the preservation of original human-written text. Implementing a watermarking standard to distinguish between human and AI-generated content could help maintain the authenticity of data used for training models. Initiatives like the Coalition for Content Provenance and Authenticity (C2PA) aim to establish guidelines for content credentialing, particularly in the case of images.
However, identifying and watermarking AI-generated text poses a greater challenge, necessitating a more thorough vetting process by AI developers. Collaborating with reputable human sources for high-quality training data may be essential to prevent the internet from being inundated with low-quality AI-generated content. By safeguarding the integrity of training data, the industry can avoid the potential consequences of model collapse and ensure the continued advancement of AI technology.
-
Destination4 months ago
Singapore Airlines CEO set to join board of Air India, BA News, BA
-
Tech News8 months ago
Bangladeshi police agents accused of selling citizens’ personal information on Telegram
-
Motivation7 months ago
The Top 20 Motivational Instagram Accounts to Follow (2024)
-
Guides & Tips6 months ago
Satisfy Your Meat and BBQ Cravings While in Texas
-
Tech News6 months ago
Soccer team’s drone at center of Paris Olympics spying scandal
-
Guides & Tips6 months ago
Have Unlimited Korean Food at MANY Unlimited Topokki!
-
Toys6 months ago
15 Best Magnetic Tile Race Tracks for Kids!
-
Breaking News5 months ago
Croatia to reintroduce compulsory military draft as regional tensions soar