Tech News
Why responsible AI is a business imperative
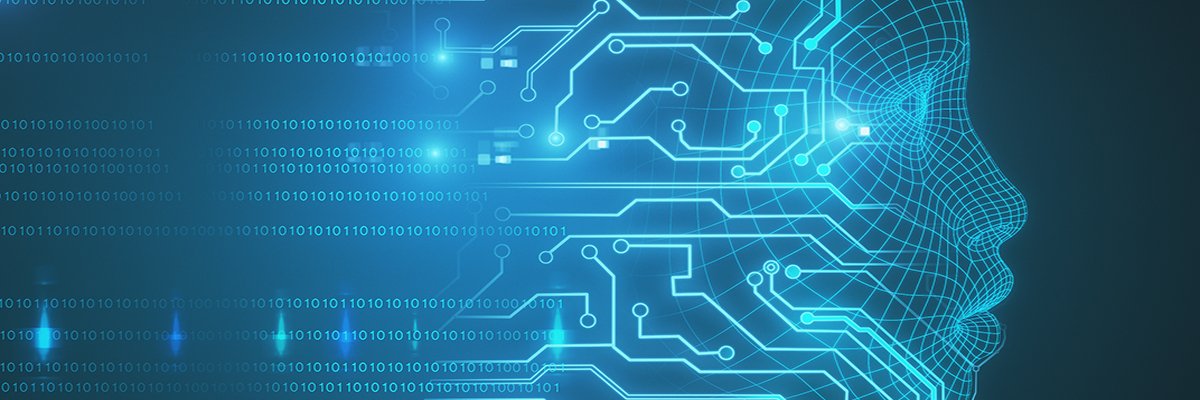
The introduction of generative artificial intelligence (AI) has sparked debates about potential AI-related harms, ranging from concerns about training data to military applications to the ethical practices of AI vendors. While critics of AI, known as “AI doomers,” have called for regulations to address long-term existential risks, they have also raised issues about current legislation focusing on competition, access, and consumer protection.
On the other hand, major AI companies like Microsoft and Google are now releasing annual transparency reports detailing how they develop and test their AI services. These reports emphasize a shared responsibility model for enterprise customers using their tools and services, similar to the approach taken in cloud security. This becomes particularly crucial as “agentic” AI tools capable of autonomous actions become more prevalent.
As AI systems, both generative and traditional, are already in use within organizations and customer-facing tools, the responsibility of AI governance needs to shift from data science teams to Chief Information Officers (CIOs). CIOs are better equipped to address AI ethics practically, considering factors such as risk tolerance, regulatory compliance, and potential impacts on business operations. According to Accenture, only a small percentage of organizations have successfully assessed risks and implemented best practices on a large scale.
Key real-world issues surrounding AI governance include lack of transparency, bias problems, accuracy issues, and challenges with defining the boundaries of AI purposes, according to Gartner analyst Frank Buytendijk. Regulations like GDPR dictate that data collected for one purpose cannot be repurposed using AI for another purpose without proper consent.
Buytendijk highlights an example: “If you are an insurance company, it is problematic to use social media data to identify smokers through AI analysis, especially if the applicants stated otherwise in their insurance application.”
Despite the looming threat of AI-specific regulations, ensuring alignment of AI tools with an organization’s core values and business objectives goes beyond mere compliance. Forrester principal analyst Brandon Purcell emphasizes the business benefits of ethical AI practices, stating that aligning AI objectives with real-world outcomes leads to increased profitability, revenue, and efficiencies.
Paula Goldman, Chief Ethical and Humane Use Officer at Salesforce, agrees, stating that building trust into AI systems enhances their effectiveness and productivity. She emphasizes the importance of AI ethics in ensuring optimal performance and productivity.
Start with principles
Responsible AI is not only a business imperative but also good business practice, as suggested by Diya Wynn, responsible AI lead at AWS. This term broadens the conversation beyond moral considerations to encompass security, privacy, and compliance perspectives necessary for organizations to address risks and unintended consequences.
Companies with compliance teams already in place for GDPR have a foundation for AI governance, although they may need to augment technical capabilities with ethical expertise in data science teams.
Responsible AI focuses on quality, safety, fairness, and reliability. Purcell advises organizations to establish a set of ethical AI principles covering accountability, competence, dependability, empathy, factual consistency, integrity, and transparency, reflecting corporate culture and values.
These principles help navigate conflicts that may arise when AI optimizations clash with different business objectives. It also provides a strong basis for implementing effective controls using tools designed for business leaders and data scientists alike. Buytendijk warns that AI ethics is a human discipline, not just a technological category.
While early responsible AI tools often prioritize technical measures like machine learning accuracy, businesses are more concerned with technosocial metrics such as balancing productivity improvements, customer satisfaction, and return on investment.
For example, generative AI chatbots may speed up call resolution but could impact overall customer satisfaction if they fail to address complex queries effectively. Custom metrics for user interaction preferences are increasingly sought after, such as measuring friendliness or the ’emotional intelligence’ of AI models.
Leading AI vendors like Microsoft, Google, Salesforce, and AWS offer emerging AI governance tools that cover various stages of the responsible AI process, from model selection to monitoring production systems.
Gateways and guardrails for genAI
Generative AI models pose unique risks compared to traditional machine learning models, requiring layered mitigations beyond fairness, transparency, and bias considerations.
Input guardrails help keep AI tools focused on specific topics, improving response accuracy and customer service. They also prevent costly multi-turn conversations that may not be resolved by the AI tool, ultimately enhancing business reputation.
Guardrails address compliance issues, ensuring that sensitive information like personally identifiable data is not processed by the AI model. Output guardrails are crucial for avoiding toxic or inaccurate responses and adhering to copyright regulations.
Azure AI Content Safety offers solutions for filtering risky content, detecting attempted breaches, and ensuring compliance with IP protections. It also addresses hallucination issues where AI generates content disconnected from available context.
Addressing hallucinations and omissions in AI responses requires grounding the model with relevant data, improving the overall quality and reliability of AI-generated content.
Handling hallucinations
Training data presents challenges for generative AI, with concerns about the legality and ethics of data scraping for large language models. Organizations can enhance AI performance by using their own data to ground generative AI models, teaching them to provide informed responses.
Techniques like Retrieval Augmented Generation (RAG) improve AI responses by incorporating information from internal data sources. Specialized domain tuning with Low-Rank Adaptation (LoRA) can enhance model performance without the high cost of full fine-tuning.
Collecting feedback is essential for improving AI systems, alongside monitoring content safety and ensuring compliance with user expectations. Feedback loops enable expert users to train AI models effectively.
Designing user experiences to facilitate natural feedback and transparent AI information presentation fosters trust and user engagement. Creating a balance between AI-generated responses and human judgment is crucial for ethical AI usage.
Goldman emphasizes the importance of “mindful friction” in user interactions to prevent unintentional biases or inappropriate actions facilitated by AI systems.
Turn training into a trump card
Considering how AI systems impact employees is essential for ethical AI implementation. Generative AI can enhance customer support by aiding employees in resolving complex issues faster, leveraging systems like RAG and domain-specific training.
Training employees in AI literacy will be a requirement under the EU AI Act, emphasizing the importance of familiarity and specific training to maximize AI tool benefits.
Empowering users to leverage AI tools efficiently and focusing on automation efficiencies rather than replacing human roles lead to improved business outcomes. Building a curriculum around AI skills enhances employee capabilities and positions the organization as a desirable employer.
Collaboration between business and development teams is crucial for effective responsible AI implementation. Governance layers and tools like impact assessment templates facilitate the integration of ethical considerations into AI projects, ensuring alignment with business goals.
Engaging a red team to stress test AI models and assess mitigation strategies allows for a comprehensive evaluation of AI readiness for production deployment. Assessing overall AI risk exposure can also highlight areas for improving data handling and governance practices.
-
Destination3 months ago
Singapore Airlines CEO set to join board of Air India, BA News, BA
-
Tech News7 months ago
Bangladeshi police agents accused of selling citizens’ personal information on Telegram
-
Motivation6 months ago
The Top 20 Motivational Instagram Accounts to Follow (2024)
-
Guides & Tips5 months ago
Have Unlimited Korean Food at MANY Unlimited Topokki!
-
Guides & Tips5 months ago
Satisfy Your Meat and BBQ Cravings While in Texas
-
Gaming4 months ago
The Criterion Collection announces November 2024 releases, Seven Samurai 4K and more
-
Self Development7 months ago
Don’t Waste Your Time in Anger, Regrets, Worries and Grudges
-
Toys6 months ago
15 of the Best Trike & Tricycles Mums Recommend