Tech News
Cloud databases: Base jumping for the bigger picture
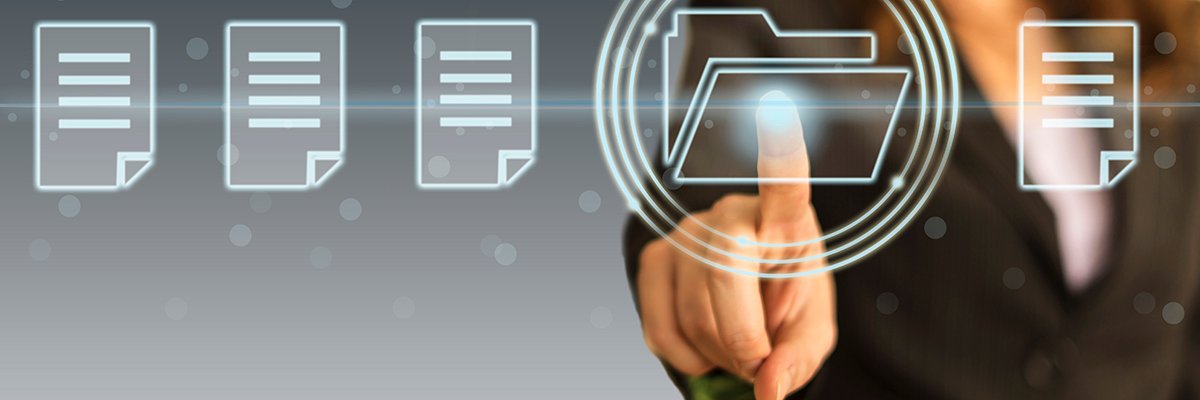
Organizations face a constant challenge in storing, managing, and analyzing data due to the overwhelming amount of data on corporate IT systems. It often feels like IT teams are playing catch-up.
Veritas reports that the average company stores around 10PB (petabytes) of data, which amounts to approximately 23 billion files. About 52% of this data is unclassified (or dark) data, and 33% is redundant, obsolete, and trivial. This not only impacts storage costs and cybersecurity but also makes analyzing and deriving insights from the data difficult. It requires a different approach to traditional data management as organizations deal with increasingly complex data relationships.
Generative artificial intelligence (GenAI) is gaining traction, especially in corporate discussions about data management. However, there is still uncertainty surrounding its practical application despite the headline-catching benefits that influence board members. According to Capgemini research, 96% of executives consider GenAI a hot topic in the boardroom, but implementing it poses challenges.
A survey by Couchbase reveals that businesses struggle with data architectures that fail to meet data demands, resulting in an average of $4 million in wasted spending. Legacy technology limitations and difficulties in accessing or managing required data are major contributors to this struggle.
Relational databases are unable to keep up with the demands of modern, data-intensive applications, causing businesses to suffer. Managing structured and unstructured datasets requires different approaches, with graph databases, a type of NoSQL database, becoming increasingly essential. The survey by Couchbase shows that many enterprises are consolidating database architectures and adopting high-performance databases to manage unstructured data efficiently.
NoSQL databases in action
Graph and other NoSQL databases are being used by various industries for their efficiency in handling interconnected data and performing complex queries with low latency. They are ideal for analyzing relationships between data points in use cases where such understanding is crucial.
Prominent users include social networks like Facebook for analyzing social graphs, financial services providers for fraud detection, and supply chain companies for optimizing logistics. NoSQL databases are widely adopted in industries such as e-commerce, IoT, and real-time analytics for managing data effectively and making quick decisions.
Scalability and flexibility
While graph databases excel at handling interconnected data and performing low-latency queries, NoSQL databases offer scalability, flexibility, and the ability to handle unstructured data in distributed environments. Teams utilize graphs for their flexibility and performance in modern data systems, making them suitable for various business domains.
Examples of organizations benefiting from graph databases include a large banking customer using it for risk profiling and Transport for London using it for road network maintenance. Another example is ExpectAI using graph database technology for climate change solutions, enabling them to navigate complex data relationships effectively.
The combination of graph technology and AI is transforming data relationships and enabling new, complex data business ideas to flourish. AI helps organize unstructured data, enabling semantic search and vector indexing for better data interpretation and insights.
The utilization of AI is set to rise as organizations seek to streamline manual processes, reduce query times, and gain deeper insights.
In the realm of AI and NoSQL, graph databases are highlighted as ideal for AI applications that involve analyzing data relationships. Advanced algorithms for tasks like recommendation systems and fraud detection benefit from the capabilities of graph databases. Bloomberg has seen success with Apache AGE, a graph database extension used for tasks ranging from data lineage tracking to deployment dashboards.
AI plays a crucial role in managing the increasing volume of structured and unstructured data needed for informed decision-making. Bloomberg continues to invest in technologies like graph and vector databases to support their comprehensive AI strategy. NoSQL databases play a vital role in AI applications, providing real-time data processing capabilities necessary for tasks like predictive maintenance and real-time analytics.
Looking ahead, the future of graph databases appears promising, with industries like healthcare, telecommunications, and finance recognizing the value of analyzing interconnected data. Cloud providers are expected to expand their database offerings, focusing on scalability and integration. The integration of AI will lead to more intelligent and data-driven applications.
Bloomberg advises organizations to experiment with use cases and leverage products like DataStax AstraDB and OpenAI to create production-ready solutions quickly. By measuring the value of these solutions early on, organizations can make informed decisions about further resource allocation.
-
Destination3 months ago
Singapore Airlines CEO set to join board of Air India, BA News, BA
-
Tech News7 months ago
Bangladeshi police agents accused of selling citizens’ personal information on Telegram
-
Motivation6 months ago
The Top 20 Motivational Instagram Accounts to Follow (2024)
-
Guides & Tips5 months ago
Have Unlimited Korean Food at MANY Unlimited Topokki!
-
Guides & Tips5 months ago
Satisfy Your Meat and BBQ Cravings While in Texas
-
Gaming4 months ago
The Criterion Collection announces November 2024 releases, Seven Samurai 4K and more
-
Self Development7 months ago
Don’t Waste Your Time in Anger, Regrets, Worries and Grudges
-
Toys6 months ago
15 of the Best Trike & Tricycles Mums Recommend